Faculty Profile
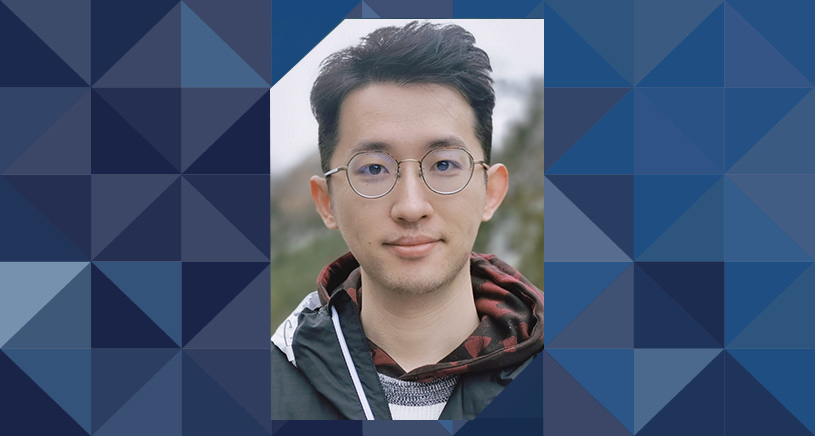
Bingkai Wang, PhD
- Assistant Professor, Biostatistics
Dr. Wang's research focuses on causal inference based on randomized trials, observational
studies, and a combination of both. He is an expert in the design and analysis of
complex clinical trials, including covariate-adaptive randomization, cluster/stepped-wedge
trials, longitudinal studies, and test-negative designs. He is interested in leveraging
modern tools, such as machine learning and large language models, to improve the practice
of clinical studies. His recent contribution is developing model-robust and efficient
causal inference methods for the above-mentioned trial designs.
- PhD, Biostatistics, Johns Hopkins University, 2021
- BS, Mathematics, Fudan University, 2016
Research Interests
I am broadly interested in causal inference, machine learning, test-negative designs in infectious-disease research, semiparametric efficiency theory, and clincal trials. In addition to methodological research, I am passionate about writing translational papers to disseminate new ideas and results to a general audience.
Research Projects
Some of my current projects include the robustness of common methods, such as linear mixed models, in complex settings of randomized trials. On top of it, I study whether machine learning can be incorporated to achieve better efficiency.
I am broadly interested in causal inference, machine learning, test-negative designs in infectious-disease research, semiparametric efficiency theory, and clincal trials. In addition to methodological research, I am passionate about writing translational papers to disseminate new ideas and results to a general audience.
Research Projects
Some of my current projects include the robustness of common methods, such as linear mixed models, in complex settings of randomized trials. On top of it, I study whether machine learning can be incorporated to achieve better efficiency.
Bingkai Wang, Chan Park, Dylan Small, and Fan Li. (2023). “Model-robust and efficient inference
for cluster-randomized experiments.” Journal of American Statistical Association:
Theory and Methods, in press.
Bingkai Wang, Ryoko Susukida, Ramin Mojtabai, Masoumeh Amin-Esmaeili, and Michael Rosenblum. (2021). “Model-Robust Inference for Clinical Trials that Improve Precision by Stratified Randomization and Adjustment for Covariate Adjustment.” Journal of American Statistical Association: Theory and Methods, 118(542): 1152-1163.
Bingkai Wang, Elizabeth L. Ogburn, and Michael Rosenblum. (2019). “Analysis of covariance in randomized trials: More precision and valid confidence intervals, without model assumptions”. Biometrics, 75(4): 1391-1400.
Bingkai Wang, Ryoko Susukida, Ramin Mojtabai, Masoumeh Amin-Esmaeili, and Michael Rosenblum. (2021). “Model-Robust Inference for Clinical Trials that Improve Precision by Stratified Randomization and Adjustment for Covariate Adjustment.” Journal of American Statistical Association: Theory and Methods, 118(542): 1152-1163.
Bingkai Wang, Elizabeth L. Ogburn, and Michael Rosenblum. (2019). “Analysis of covariance in randomized trials: More precision and valid confidence intervals, without model assumptions”. Biometrics, 75(4): 1391-1400.
Areas of Expertise: Biostatistics, Clinical Trials, Infectious Disease